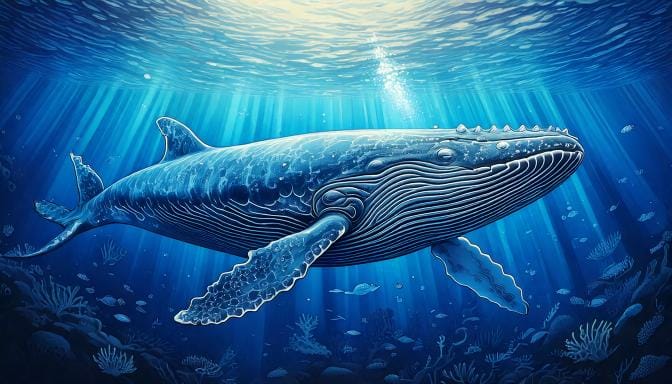
DeepSeek R1: A New Era in Open Source AI
The start of 2025 brought a groundbreaking development in artificial intelligence with DeepSeek R1, an open-source reasoning model that's reshaping the AI landscape. Using innovative MoE architecture and novel training approaches, it offers unprecedented accessibility and performance.
The start of 2025 brought a groundbreaking development in artificial intelligence with the release of DeepSeek R1, an open-source reasoning model that's reshaping the AI landscape. Let's explore what makes this model special and how it could impact the future of AI development.
Key Takeaways
- DeepSeek R1 is an open-source AI model released under the MIT license
- The model uses innovative MoE architecture, activating only 37B out of 671B parameters per task
- Training costs are 95% lower than competing models
- API costs are significantly reduced at $0.55 per million tokens
- Distilled versions can run on consumer GPUs while maintaining high performance
Open Source and Accessibility
DeepSeek R1's open-source nature marks a significant shift in AI accessibility. Released under the MIT license, both the technology and research are publicly available. The transparency extends to their research paper and replication project, allowing developers and researchers to build upon their work.
What sets DeepSeek R1 apart is its cost-effectiveness. The model achieves a remarkable 95% reduction in training costs compared to competitors, and its API pricing at $0.55 per million tokens makes it significantly more affordable for real-world applications. This dramatic cost reduction opens up possibilities for businesses of all sizes to implement advanced AI capabilities.
Architecture and Innovation
The model employs a Mixture-of-Experts (MoE) architecture, demonstrating remarkable efficiency. Out of its total 671 billion parameters, DeepSeek R1 activates only 37 billion parameters for each specific task. This selective activation ensures optimal performance while maintaining efficiency, allowing the model to adapt to different types of problems without unnecessary computational overhead.
Training Approach
DeepSeek R1 introduces a novel training methodology that prioritizes Reinforcement Learning (RL) over traditional Supervised Fine-Tuning (SFT). The training process consists of several key components:
- Pure RL Training (DeepSeek-R1-Zero):
- Self-learning framework
- Development of complex reasoning capabilities
- Chain-of-Thought reasoning implementation
- Enhanced Training Pipeline:
- Cold start with curated datasets
- Generalized Reward-Policy Optimization (GRPO)
- Multi-stage reinforcement learning
- Continuous refinement and validation
Practical Applications
The accessibility and efficiency of DeepSeek R1 open up numerous possibilities for developers and businesses:
- Local Deployment: Distilled versions (1.5B to 70B parameters) can run on consumer hardware
- Cost-Effective API Integration: Significantly lower API costs enable broader implementation
- Research and Development: Open-source nature facilitates innovation and improvement
Future Implications
DeepSeek R1's release could mark a turning point in AI development, making advanced AI capabilities more accessible to developers and businesses worldwide. Its combination of open-source accessibility, cost-effectiveness, and innovative architecture sets a new standard for future AI models.
Conclusion
DeepSeek R1 represents a significant step forward in democratizing AI technology. Its open-source nature, coupled with groundbreaking architecture and training methodologies, makes it a compelling option for developers and organizations looking to implement advanced AI capabilities without excessive costs or resource requirements.
In our next article, we'll explore how we can use deepseek in our Spring Environment.